Summary
For the sub-health population, people can not only reduce the risk of disability, but avoid the occurrence of other diseases if diseases status could be detected in the pre-clinical stage, and accordingly, risk factors could be managed. According to WHO GBD 2016 analysis, non-communicable diseases such as metabolic diseases (diabetes, obesity, osteoporosis), cardiovascular diseases (coronary artery calcification, atrial fibrillation, hypertension), lung diseases (pulmonary nodules/lung cancer, chronic obstructive pulmonary disease), etc. lead to more than 70% of mortality. The public health burden impact of chronic disease is increasing. Compared with traditional statistical prediction models, artificial intelligence (AI) risk prediction models could be better in combining different and multiple data to achieve better risk predictions.
This project aims to apply AI and machine learning techniques on building risk prediction models of chronic diseases, which would be trained and validated using the longitudinal data for sub-health population in hope to improve the model accuracy.
This project used the Integrated Medical Database of National Taiwan University Hospital (NTUH-iMD) from 2006 to 2017. The database provided clinical records from outpatient, inpatient, and emergency departments. The Department of Pharmacy supported the prescription data, and the Department of Laboratory Medicine provided results of laboratory examinations.
It is anticipated that prediction models with AI techniques can improve the accuracy, and subsequently, clinical decision making for medical care of chronic disease among sub-health population.
Advantages
AI and machine learning techniques on the longitudinal data for sub-health population provide alternative ways to establish risk prediction models, thus improving model the prediction accuracy of traditional prediction models.
Applications
For the insurance industry, risk prediction model can offer insurance advice and information. This model could be used to facilitate individualized decision making, and to reduce the cost of primary prevention.
For health fitness industry, risk prediction models could be used to provide risk stratifications, thereby lifestyle modifications and customized exercise program could be suggested.
Keywords
Chronic disease, risk prediction model, machine learning, sub-health population
◎ PI
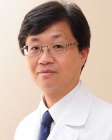
PI Han-Mo Chiu
Clinical Professor, Dept. of Internal Medicine, College of Medicine, NTU
Director, HMC, NTUH