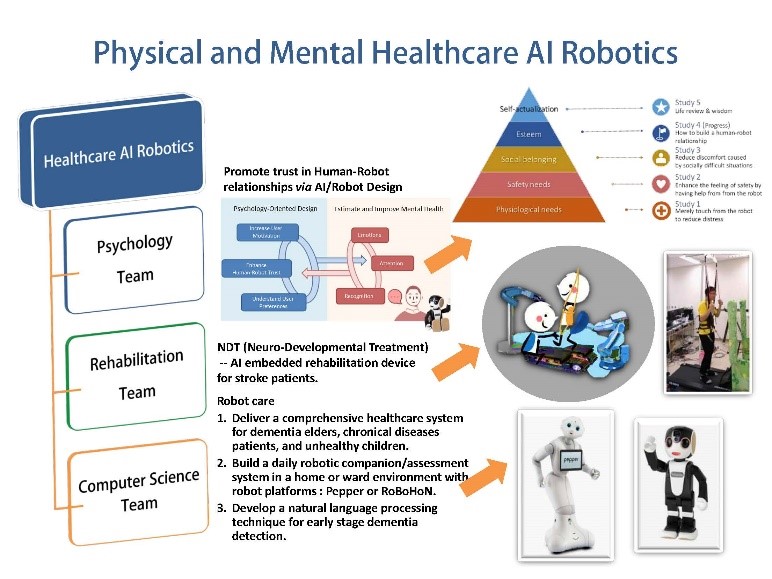

Summary
In this project, we develop an all-round AI robotic system that cares physical and mental states of the elders and those who need rehabilitation. The project runs with two major themes: 1). Our robotic system functions as a three-phase caring system based on AI technologies, which deals with early detection, midterm intervention/therapy, and later rehabilitation on elders hit by the chronic illness or suffering from stroke or dementia; 2). To improve the user experience of our AI healthcare system, we integrate the knowledge of psychology and AI to construct a humanized AI system that achieves mental care. The research topics conducted in this project are around humanized design and start with the following three aspects, namely, memory assistance, emotional soothing, and reliable relation. We investigate the AI technologies, including the customized natural language process, emotion expression, company, self-care system, and cross-sense information integration system.
Applications
In this project, we develop an all-round AI robotic system that cares physical and mental states of the elders and those who need rehabilitation. The project runs with two major themes: 1). Our robotic system functions as a three-phase caring system based on AI technologies, which deals with early detection, midterm intervention/therapy, and later rehabilitation on elders hit by the chronic illness or suffering from stroke or dementia; 2). To improve the user experience of our AI healthcare system, we integrate the knowledge of psychology and AI to construct a humanized AI system that achieves mental care. The research topics conducted in this project are around humanized design and start with the following three aspects, namely, memory assistance, emotional soothing, and reliable relation. We investigate the AI technologies, including the customized natural language process, emotion expression, company, self-care system, and cross-sense information integration system.
Applications
- Machine-assisted automatic physical therapy.
- Automated, non-obtrusive diagnosis of mental afflictions through audiovisual cues.
- Deployment of clinical mental care interventions using robots as a medium.
- Development of robot behaviors and conducts to promote their acceptance and integration into society.
Advantages
- Manpower shortages in the service and healthcare industries are projected to decrease globally, particularly in developed countries, due to super-aging societies. Socially assistive robots are a solution to this shortcoming.
- Physical rehabilitation therapy requires specialized trainers. Robots can be programmed to execute a routine with little effort. Furthermore, they do not feel fatigued and have been shown to be more effective than human trainers.
- Although there may exist precise methods to determine the degree of deterioration of the brain through complex examinations, we aim to develop automated, non-obtrusive techniques that can enable a robot to estimate the state of the user through continuous observation and evaluation.
- As the State-of-the-Art in AI Robotics progresses, the role of robots in the healthcare industry can change from service to a companion that can offer social and emotional support to its users.
- We perform a comprehensive evaluation of the usability and impact of our therapy tools, both from a technical and a psychological viewpoint.
Keywords
Socially Assistive Robotics, Robot-Assisted Therapy, Automatic Mental Health Diagnosis and Tracking
◎ PI
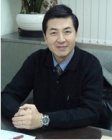
PI Li-Chen Fu
Chair Professor, Department of CSIE&EE, NTU / Director, AIROBO, NTU
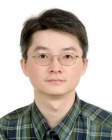
Co-PI Pei-Chun Lin
Professor, Department of Mechanical Engineering, NTU
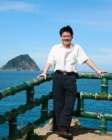
Co-PI Chung-Huang Yu
Associate Professor, Department of Physical Therapy and Assistive Technology, NYMU
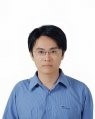
Co-PI Hung-Yi Lee
Associate professor, Department of Electrical Engineering, NTU
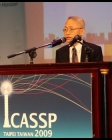
Co-PI Lin-Shan Lee
Distinguished Professor, Department of Electrical Engineering, NTU
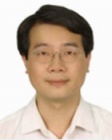
Co-PI Fu-Cheng Wang
Professor, Department of Mechanical Engineering, NTU
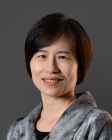
Co-PI Su-Ling Yeh
Distinguished Professor, Department of Psychology, NTU
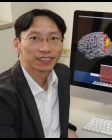
Co-PI Joshua Oon Soo Goh
Associate Professor, Graduate Institute of Brain and Mind Sciences, NTU
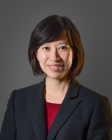
Co-PI Yu-Ling Chang
Associate Professor, Department of Psychology, NTU
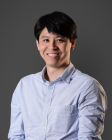
Co-PI Tsung-Ren (Tren) Huang
Associate Professor, Department of Psychology, NTU
[June 11th, 2021] Paper accepted by international journal Sensors !
Detection and Classification of Stroke Gaits by Deep Neural Networks Employing Inertial Measurement Units
Fu-Cheng Wang, Szu-Fu Chen, Chin-Hsien Lin, Chih-Jen Shih, Ang-Chieh Lin, Wei Yuan, You-Chi Li and Tien-Yun Kuo
Sensors 2021, 21, 1864. https://doi.org/10.3390/s21051864
(This is an Open Access paper, click the link to read fulltext for free.)
This paper develops Deep Neural Network (DNN) models that can recognize stroke gaits. Stroke patients usually suffer from partial disability and develop abnormal gaits that can vary widely and need targeted treatments. Evaluation of gait patterns is crucial for clinical experts to make decisions about the medication and rehabilitation strategies for the stroke patients. However, the evaluation is often subjective, and different clinicians might have different diagnoses of stroke gait patterns. In addition, some patients may present with mixed neurological gaits. Therefore, we apply artificial intelligence techniques to detect stroke gaits and to classify abnormal gait patterns. First, we collect clinical gait data from eight stroke patients and seven healthy subjects. We then apply these data to develop DNN models that can detect stroke gaits. Finally, we classify four common gait abnormalities seen in stroke patients. The developed models achieve an average accuracy of 99.35% in detecting the stroke gaits and an average accuracy of 97.31% in classifying the gait abnormality. Based on the results, the developed DNN models could help therapists or physicians to diagnose different abnormal gaits and to apply suitable rehabilitation strategies for stroke patients.
Detection and Classification of Stroke Gaits by Deep Neural Networks Employing Inertial Measurement Units
Fu-Cheng Wang, Szu-Fu Chen, Chin-Hsien Lin, Chih-Jen Shih, Ang-Chieh Lin, Wei Yuan, You-Chi Li and Tien-Yun Kuo
Sensors 2021, 21, 1864. https://doi.org/10.3390/s21051864
(This is an Open Access paper, click the link to read fulltext for free.)
This paper develops Deep Neural Network (DNN) models that can recognize stroke gaits. Stroke patients usually suffer from partial disability and develop abnormal gaits that can vary widely and need targeted treatments. Evaluation of gait patterns is crucial for clinical experts to make decisions about the medication and rehabilitation strategies for the stroke patients. However, the evaluation is often subjective, and different clinicians might have different diagnoses of stroke gait patterns. In addition, some patients may present with mixed neurological gaits. Therefore, we apply artificial intelligence techniques to detect stroke gaits and to classify abnormal gait patterns. First, we collect clinical gait data from eight stroke patients and seven healthy subjects. We then apply these data to develop DNN models that can detect stroke gaits. Finally, we classify four common gait abnormalities seen in stroke patients. The developed models achieve an average accuracy of 99.35% in detecting the stroke gaits and an average accuracy of 97.31% in classifying the gait abnormality. Based on the results, the developed DNN models could help therapists or physicians to diagnose different abnormal gaits and to apply suitable rehabilitation strategies for stroke patients.
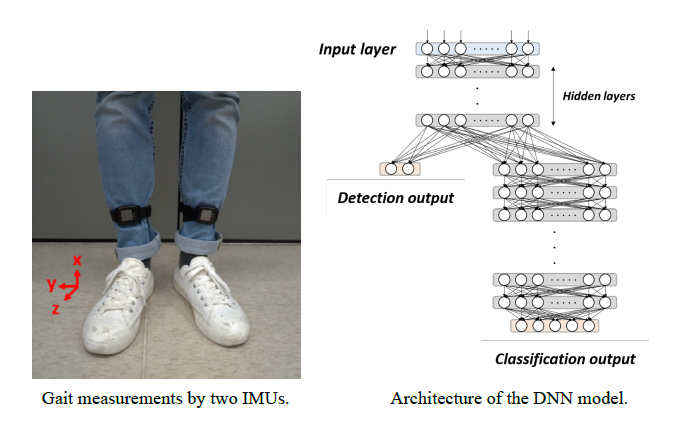
